Artificial Intelligence (AI) in Medical Education: Maintaining Human-Centeredness
Picture this: you are a first-year medical student preparing for a major exam, beginning to see why medical learning can feel like “drinking from a fire hose” – the volume of material can be overwhelming! But then you wonder: could artificial intelligence (AI) help you funnel that water into manageable buckets? Imagine an AI tool that organizes your scattered class notes and lectures into personalized flashcards, targeting your strengths and weaknesses.
Now, consider being a third-year medical student on clinical rotations, receiving written evaluations of your performance. Amidst paragraphs describing your clinical strengths and suggestions for improvement, you wonder if there are common themes across your evaluators’ comments. Could AI distill recurring themes into clear bullet points, helping you focus and improve on the wards?
Or perhaps you are a busy faculty member writing a letter of recommendation for a student you have previously evaluated. Could AI analyze your past feedback and generate a draft, saving you precious time while maintaining the letter’s quality?
Across all stages of medical education, AI has generated curiosity, excitement, and debate. But how do learners, trainees, and educators envision AI’s role in their training? Where could it provide the most value, and how can the next generation of physicians be equipped to use this technology responsibly and effectively?
Student and Trainee Perspectives
Andrew Rosenblatt, an MS1, believes that AI can “enhance, rather than replace” core skills in medical education while fostering the development of new ones.
“Platforms like UCSF Versa can enhance clinical reasoning by generating illness scripts or differential diagnoses, allowing students to focus on honing their critical thinking skills,” he explains. UCSF Versa is a generative AI platform approved for safe use with UCSF data, unlike similar platforms that are not compliant with HIPAA protections.
However, Rosenblatt acknowledges challenges of AI integration. “Overreliance on AI could weaken foundational knowledge and clinical intuition, and biases in AI systems risk reinforcing health care inequities,” he notes. “Adapting to these tools requires a learning curve for both students and educators.”
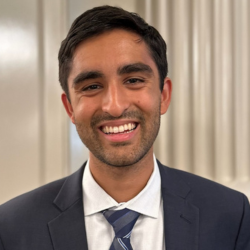
Alex Silva, a fifth-year student in the Medical Scientist Training Program, sees AI as particularly beneficial for visual-based fields like pathology and radiology, where subtle details can be difficult for untrained students to discern.
“AI can be used to annotate different findings on radiological images, which students could use as learning aids,” Silva explains. These aids could complement and contextualize the limited examples shown in class lectures.
In research, AI has the potential to uncover nuances in data that a human might miss. As a member of the Chang Lab – whose work has been extensively covered by The New York Times, The Wall Street Journal, and NPR – Silva has witnessed this phenomenon firsthand. Using brain-computer interfaces that rely on AI, Silva and his colleagues “decode brain signals into intended movements or speech for people who are severely paralyzed.” As Silva explains, “These devices may soon become used in clinical practice to restore function to people who are paralyzed due to stroke or neurodegenerative disease.
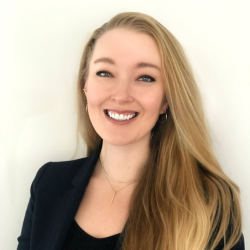
In the hospital, Diana Funk, MD, MBA, Chief Resident in Internal Medicine, sees AI as a tool to streamline “rote, high-effort, low-yield tasks,” allowing more time for patient care and medical student and resident teaching.
“Could AI alert me about the patient’s overnight fever first thing in the morning when I log in to pre-round?” Dr. Funk suggests. “Could AI suggest follow-up labs for a patient’s new anemia?”
For Dr. Funk, the potential to save time through AI-streamlined tasks is “exciting,” but challenges remain. “Right now, we have a lot of tools without much of an instruction manual,” she says. “It will be important to proactively identify what role we want AI to play, instead of throwing cool, exciting, flashy spaghetti at the wall.”
Approach with Caution and Maintain Human-Centeredness
This proactive approach marks a pivotal moment in our relationship with AI.
Where AI has become ubiquitous, it is often used passively. Tools like autocorrect, Zoom’s closed captioning, and mobile navigation apps are so ingrained in daily life that we rarely double-check them. At worst, this reliance could potentially lead to harm, as seen in a tragic case wherein a navigation app allegedly directed a driver onto a collapsed bridge. These incidents underscore the need for proactive human oversight of AI.
Another concern is the “black box problem”: our limited understanding of how AI makes its decisions. This is particularly troubling in medicine, where a student’s step-by-step clinical reasoning is essential to both patient care and teaching. Clinical instructors use students’ reasoning to evaluate knowledge and provide guidance. But if AI’s decision-making process is opaque – even to its developers – then how can it be evaluated, improved, or trusted to meet the highest standards of patient care?
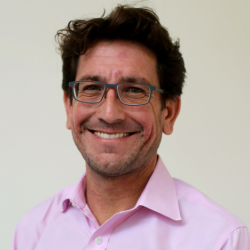
For Chandler Mayfield, Executive Director of Technology Enhanced Education at the UCSF School of Medicine, the solution begins with increasing AI literacy among faculty, learners, and staff. He emphasizes the importance of understanding “how these technologies work” and addressing the biases inherent in the training data used by AI models.
As Silva explains, “AI algorithms are limited by the breadth and diversity of training data that they see. Thus, if algorithms are trained on a biased set of data, they will have similar biases and limitations when used.”
In other words, if its training data is biased, then the algorithm will replicate those biases. One of the most well-known examples of such bias is the underperformance of AI models in diagnosing skin disease on darker skin tones compared to lighter ones. This underperformance is often attributed to the lack of diverse skin tone representation in dermatology education materials, and subsequently, in AI training data sets.
Therefore, Silva advocates for incorporating “a course on the limitations of AI” into medical education. Such a course would teach students to think critically about whether a specific AI tool is appropriate for a given context. Similarly, Rosenblatt believes such courses could teach students to recognize and address inequities in their education and practice. In his view, AI could “democratize access to quality educational resources” and bridge gaps for students in underserved areas.
Dr. Funk believes that an AI curriculum should emphasize the core skills that are essential to evidence-based medicine. “Incorporating AI necessitates education about interpreting data… and critical appraisal of sources and outcomes,” she explains, noting that such skills are as relevant to evaluating landmark drug trials as they are to assessing AI-generated outputs or screening recommendations.
But opinions on AI in medical education vary widely, which Mayfield notes is typical during major technological disruptions. “Some faculty are concerned that AI tools may undermine the teaching and learning experience of core skills, such as critically appraising the literature or developing effective study habits,” he says. Other faculty want learners to use AI in order to investigate pitfalls like “hallucinations,” in which AI generates false or misleading information.
But even if all these issues are resolved, one key consideration remains: to ensure a human-centered approach that maintains the trust and empathy inherent to patient care.
“It’s crucial to maintain a focus on the human connection and interpersonal skills that are at the heart of medicine,” says Rosenblatt. “UCSF has taught us that we see these technologies as a complement to, not a replacement for, the critical thinking and empathy that define great physicians.”
Mayfield agrees. “The core focus of medicine and our missions at UCSF is human-centered,” he says. “We should always remain focused on how these tools should improve the lived experiences of our people and patients and the public.”
Collaboration in the Face of an Uncertain Future: the UCSF AI and Education Symposium
The future of AI in medical education remains uncertain. What is clear, however, is that the rapid rise of AI has outpaced the ability of academic curricula to effectively integrate these technologies.
For Mayfield, this presents both a challenge and an opportunity: to prepare graduates to use AI tools ethically, effectively, and responsibly in their daily work to ensure the best outcomes.
UCSF faculty, staff, and learners are already advancing AI in the classroom, clinic, and hospital. To meet the growing demand for clinicians trained in AI, UCSF has also expanded educational programs and certificates focused on AI development and application.
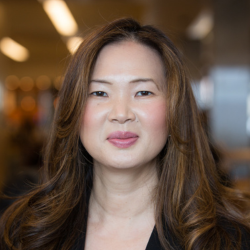
Leading these efforts is Christy Boscardin, PhD, Professor of Medicine, who was recently named Director of AI and Assessment for Medical Education. Boscardin will spearhead initiatives to integrate AI into teaching, learning, assessment, and administrative processes, leveraging its capabilities to enhance current practices.
“As AI becomes more prevalent, it’s essential that the UCSF medical education community come together to help lead how these tools should be implemented,” said Dr. Boscardin. “If we keep our focus on people and solving meaningful problems, I believe AI can benefit learners and educators while improving the patient experience.”
Engaging the entire UCSF medical education community will be essential in shaping the role of AI. To foster collaboration, the UCSF AI and Education Symposium on February 12, 2025, will bring together perspectives from all UCSF professional schools to refine a shared vision for AI in medical education.
A Roadmap for Next Steps
AI is not the future – it is already here and has been for decades. Its integration into medical education raises more questions than answers, particularly around learning methodologies, patient privacy, safety, data bias, and the risks of false or confabulated information.
But whether generating flashcards or recommendation letters, annotated images or automated alerts, one thing is clear: as AI evolves, we must actively validate every aspect of its development, outputs, and applications. We must scrutinize its data sources, critically appraise outcomes, and foster cautious, informed engagement over passive reliance.
In doing so, we may begin to foster truly human-centered AI tools that benefit our learners – and the patients they will serve in the future.